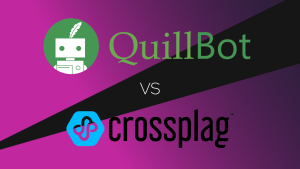
QuillBot vs. CrossPlag: A Comprehensive Comparison
In the rapidly evolving landscape of artificial intelligence (AI) and digital content creation, tools like QuillBot and CrossPlag have become essential for various user needs.
What AI Detector is Always True? The Reliability of AI
Detection Technology
Content writer and editor for Netus.AI
What AI Detector is Always True? AI detectors have become increasingly popular in recent years, with their ability to detect and analyze patterns and anomalies in data. However, the accuracy of these detectors can vary widely depending on the technology used and the specific application. As such, the question arises: what AI detector is always true?
While it may be tempting to assume that there is a single AI detector that is always accurate, the reality is that the answer is more complex than that. Different AI detectors are designed for different purposes, and their accuracy can vary depending on the specific task at hand. For example, an AI detector designed to identify faces may be highly accurate in that specific task, but may not perform as well when tasked with identifying objects or animals.
That being said, there are certain characteristics that can make an AI detector more accurate overall. These include the quality of the data used to train the detector, the complexity of the algorithms used, and the ability of the detector to adapt to new data and situations. By carefully evaluating these factors, it is possible to identify AI detectors that are more likely to be accurate and reliable in a variety of applications.
AI detectors are algorithms that are designed to identify and classify specific patterns or behaviors in data. These detectors are used in a wide range of applications, from fraud detection to image recognition. They are particularly useful in situations where large amounts of data need to be analyzed quickly and accurately.
AI detectors are typically based on machine learning algorithms that are trained on large datasets of labeled data. During the training process, the algorithm learns to recognize specific patterns or behaviors in the data, and can then apply that knowledge to new, unseen data.
One of the key advantages of AI detectors is their ability to learn and adapt over time. As new data becomes available, the algorithm can be retrained to improve its accuracy and performance.
Machine learning plays a crucial role in the development and deployment of AI detectors. By leveraging large datasets and powerful algorithms, machine learning enables AI detectors to identify patterns and behaviors that would be difficult or impossible for humans to detect.
However, it is important to note that AI detectors are only as good as the data they are trained on. If the training data is biased or incomplete, the detector may produce inaccurate or unreliable results.
In conclusion, AI detectors are powerful tools that can be used to identify and classify specific patterns or behaviors in data. By leveraging machine learning algorithms and large datasets, these detectors can provide accurate and reliable results in a wide range of applications. However, it is important to ensure that the training data is unbiased and complete in order to achieve the best possible results.
The accuracy and precision of an AI detection method are crucial factors in determining its reliability. Accuracy refers to the percentage of correct detections out of all detections made, while precision refers to the percentage of correct detections out of all positive detections made.
A high accuracy and precision rate is desirable, as it indicates that the AI detector is consistently making correct detections. However, it is important to note that a high accuracy rate does not necessarily mean a high precision rate, and vice versa. Therefore, it is important to evaluate both accuracy and precision when assessing the effectiveness of an AI detection method.
False positives and false negatives are errors that can occur in AI detection methods. False positives occur when the detector incorrectly identifies something as positive, while false negatives occur when the detector fails to identify something as positive.
The false positive rate and false negative rate are important metrics to consider when evaluating an AI detection method. A low false positive rate and false negative rate indicate that the detector is making fewer errors and is thus more reliable.
Perplexity and burstiness metrics are used to evaluate the performance of language models in AI detection methods. Perplexity measures how well a language model can predict the next word in a sentence, while burstiness measures the frequency of certain words or phrases in a given context.
A lower perplexity score indicates that the language model is better at predicting the next word in a sentence, while a lower burstiness score indicates that the language model is better at detecting unusual or unexpected language patterns.
In conclusion, evaluating AI detection methods involves considering factors such as accuracy, precision, false positive and false negative rates, as well as perplexity and burstiness metrics. By assessing these metrics, one can determine the reliability and effectiveness of an AI detection method.
Plagiarism is a serious academic offense that can result in severe consequences for students. To prevent academic dishonesty, many universities and academic institutions use plagiarism checkers that utilize AI technology. These checkers scan student papers and compare them to a vast database of academic works to detect any instances of plagiarism.
AI-powered plagiarism checkers can detect even minor instances of plagiarism, making them an effective tool for maintaining academic integrity. They can also help students avoid accidental plagiarism by highlighting any areas where their writing may be too similar to existing works.
AI writing detection is another technology used in academic settings to maintain academic integrity. This technology uses AI algorithms to analyze student writing and detect any instances of ghostwriting or outsourced work.
By using AI writing detection, academic institutions can ensure that students are producing their own work and not relying on ghostwriters or other sources. This technology can also help students improve their writing skills by highlighting areas where they may need additional support.
Overall, AI detection is an essential tool for maintaining academic integrity in academic settings. By utilizing plagiarism checkers and AI writing detection, universities and academic institutions can ensure that students are producing original work and upholding academic standards.
One of the biggest challenges of AI detectors is dealing with evolving AI models. As new data becomes available, AI models must be updated to ensure that they remain accurate and effective. However, updating AI models can be a complex and time-consuming process. It requires a significant amount of data, as well as expertise in machine learning and data analysis.
Furthermore, updating AI models can also lead to new challenges. For example, it can be difficult to ensure that the updated model is still reliable and trustworthy. Additionally, updating AI models can sometimes introduce bias, which can lead to false positives or false negatives.
Another challenge of AI detectors is addressing bias and reliability issues. AI detectors are only as reliable as the data they are trained on. If the data is biased, the AI detector will also be biased. This can lead to false positives or false negatives, which can have serious consequences.
To address bias and reliability issues, it is important to ensure that the data used to train AI detectors is diverse and representative. This can be done by using data from a variety of sources and by including a diverse range of examples in the training data. It is also important to regularly evaluate the performance of AI detectors to ensure that they remain reliable and trustworthy.
Overall, while AI detectors have the potential to be highly effective, they also face a number of challenges and limitations. To address these challenges, it is important to continually monitor and evaluate the performance of AI detectors, and to take steps to address any issues that arise. By doing so, it is possible to ensure that AI detectors remain reliable and trustworthy tools for a variety of applications.
As AI technology continues to evolve, so do the algorithms used for detection. AI content detectors are becoming more sophisticated and accurate, allowing them to identify even the most subtle signs of potential threats. This means that AI detection accuracy is expected to improve significantly in the coming years, making it an even more valuable tool for security and safety.
One of the most exciting developments in AI detection technology is the use of machine learning algorithms. These algorithms are designed to learn and adapt over time, allowing them to improve their accuracy and effectiveness as they process more data. This means that AI systems will become more intelligent and efficient at detecting potential threats, making them an even more valuable tool for security and safety.
The development of new AI systems is expected to have a significant impact on detection technology. For example, AI systems that are designed to work in conjunction with human operators will make it easier for security personnel to identify and respond to potential threats. Additionally, AI systems that are designed to work in real-time will make it possible to detect threats more quickly, allowing for a faster response time.
Another important aspect of new AI systems is their ability to process large amounts of data quickly and accurately. This means that they will be able to analyze vast amounts of information in real-time, allowing them to identify potential threats and respond to them quickly and effectively.
Overall, the future of AI detection technology looks bright. With advancements in detection algorithms and the development of new AI systems, AI technology will become an even more valuable tool for security and safety. As AI systems become more intelligent and efficient, they will be able to detect potential threats more quickly and accurately, allowing for a faster response time and better overall security.
In the rapidly evolving landscape of artificial intelligence (AI) and digital content creation, tools like QuillBot and CrossPlag have become essential for various user needs.
AI-generated content has surged, making tools like Quillbot and Originality.AI indispensable for writers, educators, and content creators.
In short Yes, Turnitin can detect Deepl translated text. The rise of AI-driven tools in academia, such as DeepL for translation and Turnitin for plagiarism detection, has sparked significant debate.
Turnitin is a widely used tool in educational institutions to check for plagiarism by comparing submitted work against an extensive database of academic papers, websites, and other content.
In the rapidly evolving world of artificial intelligence tools for academia and content creation, it’s crucial to understand the differences between platforms.
Welcome to the world of plagiarism detection tools! In this article, we will compare two popular options: Originality.ai and Turnitin
@ 2024 Netus AI.